Boardgames in the Commons
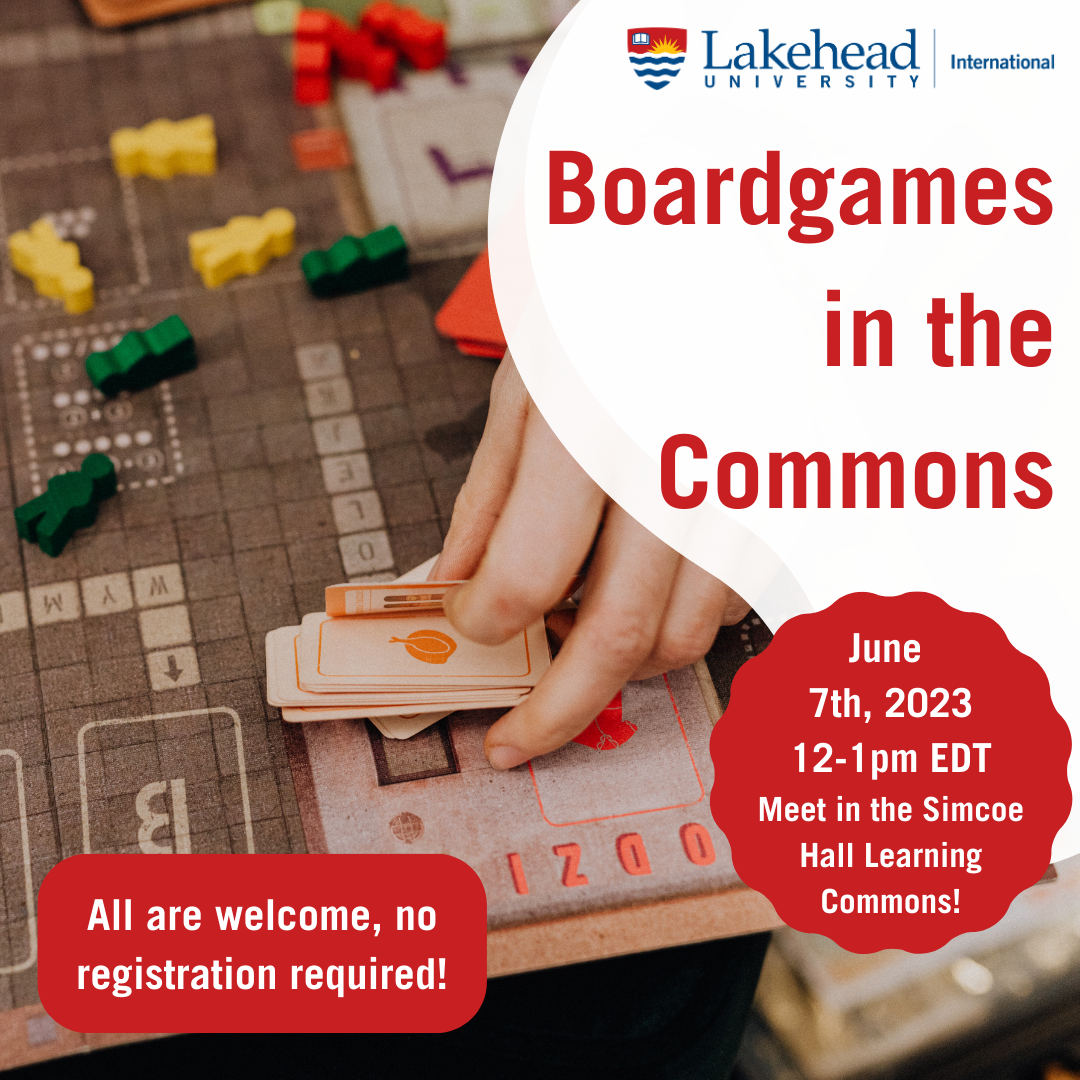
Take a break to connect with peers over some fun games! All are welcome, no registration required.
Take a break to connect with peers over some fun games! All are welcome, no registration required.
The math competency exam is for students entering Year 1 of the two-year Bachelor of Education professional program. Students will receive room allocations from the Education Programs Officer, Julie Howell, this summer.
Thunder Wolf Racing invites you to attend the unveiling for TWR-15, our 2022-2023 car. The unveiling will take place at the Lakehead University campus Lot 6 on May 12th at noon. Come meet our team and see the car we've worked so hard on the past 8 months to design and build before we depart for the Michigan International Speedway. All are welcome to attend.
Join us for some fun outdoor games while socializing with peers! No skill required.
Join us for a leisurely walk on a local trail! No hiking skills required. Please don't forget to bring a water bottle, hat/sunglasses, and wear closed-toe shoes.
The Biotechnology PhD candidate, Niravkumar Kosamia will present his research: Multicriteria Feasibility Assessment of BioSuccinic Acid Production from Lignocellulosic Biomass
May 10, 2023
1:00 pm
FB 2023 and Zoom
Committee Members:
Drs. Sudip Rakshit and Arturo Sánchez Carmona (co-supervisors), Dr. Baoqiang Liao, Dr. Siamak Elyasi
and Dr. Vijai Kumar Gupta (external)
Everyone is welcome
For more information contact Brenda Magajna at phd.ses@lakeheadu.ca
Please join the Computer Science Department for the upcoming thesis defense:
Presenter: Jingtian Zhao
Thesis title: Adding Time-series Data to Enhance Performance of Natural Language Processing Tasks
Abstract: In the past few decades, with the explosion of information, a large number of computer scientists have devoted themselves to analyzing collected data and applying these findings to many disciplines. Natural language processing (NLP) has been one of the most popular areas for data analysis and pattern recognition. A significantly large amount of data is obtained in text format due to the ease of access nowadays. Most modern techniques focus on exploring large sets of textual data to build forecasting models; they tend to ignore the importance of temporal information which is often the main ingredient to determine the performance of analysis, especially in the public policy view. The contribution of this paper is three-fold. First, a dataset called COVID-News is collected from three news agencies, which consists of article segments related to wearing masks during the COVID-19 pandemic. Second, we propose a long-short term memory (LSTM)-based learning model to predict the attitude of the articles from the three news agencies towards wearing a mask with both temporal and textural information. Then we added the BERT model to further improve and enhance the performance of the proposed model. Experimental results on the COVID-News dataset show the effectiveness of the proposed LSTM-based algorithm.
Committee Members:
Dr. Yimin Yang (supervisor, committee chair), Dr. Ruizhong Wei (co-supervisor), Dr. Amin Safaei, Dr. Thangarajah Akilan (Software Engineering)
Please contact grad.compsci@lakeheadu.ca for the Zoom link.
Everyone is welcome.
Please join the Computer Science Department for the upcoming thesis defense:
Presenter: Weiting Liu
Thesis title: Vapnik-Chervonenkis Dimension in Neural Networks
Abstract: This academic article aims to explore the potential of statistical concepts, specifically the Vapnik-Chervonenkis Dimension (VCD), in optimizing neural networks. With the increasing use of neural networks and machine learning in replacing human labor, ensuring the safety and reliability of these systems is a critical concern.
The article delves into the question of how to test the safety of neural networks and optimize them through accessible statistical concepts. The article presents two case studies to demonstrate the effectiveness of using VCD in optimizing neural networks. The first case study focuses on optimizing the autoencoder, a neural network with both encoding and decoding functions, through the calculation of the VC dimension. The conclusion suggests that optimizing the activation function can improve the accuracy of the autoencoder at the mathematical level.
The second case study explores the optimization of the VGG16 neural network by comparing it to VGG19 in terms of their ability to process high-density data. By adding three hidden layers, VGG19 outperforms VGG16 in learning ability, suggesting that adjusting the number of neural network layers can be an effective way to optimize neural networks.
Overall, this article proposes that statistical concepts such as VCD can provide a promising avenue for optimizing neural networks, thus contributing to the development of more reliable and efficient machine learning systems. The final vision is to allocate the mathematical model reasonably to machine learning and establish an idealized neural network establishment, allowing for safe and effective use of neural networks in various industries.
Committee Members:
Dr. Yimin Yang (supervisor, committee chair), Dr. Amin Safaei, Dr. Fang (Fiona) Fang (Western University)
Please contact grad.compsci@lakeheadu.ca for the Zoom link.
Everyone is welcome.
Please join the Computer Science Department for the upcoming thesis defense:
Presenter: Weiting Liu
Thesis title: Vapnik-Chervonenkis Dimension in Neural Networks
Abstract: This academic article aims to explore the potential of statistical concepts, specifically the Vapnik-Chervonenkis Dimension (VCD), in optimizing neural networks. With the increasing use of neural networks and machine learning in replacing human labor, ensuring the safety and reliability of these systems is a critical concern.
The article delves into the question of how to test the safety of neural networks and optimize them through accessible statistical concepts. The article presents two case studies to demonstrate the effectiveness of using VCD in optimizing neural networks. The first case study focuses on optimizing the autoencoder, a neural network with both encoding and decoding functions, through the calculation of the VC dimension. The conclusion suggests that optimizing the activation function can improve the accuracy of the autoencoder at the mathematical level.
The second case study explores the optimization of the VGG16 neural network by comparing it to VGG19 in terms of their ability to process high-density data. By adding three hidden layers, VGG19 outperforms VGG16 in learning ability, suggesting that adjusting the number of neural network layers can be an effective way to optimize neural networks.
Overall, this article proposes that statistical concepts such as VCD can provide a promising avenue for optimizing neural networks, thus contributing to the development of more reliable and efficient machine learning systems. The final vision is to allocate the mathematical model reasonably to machine learning and establish an idealized neural network establishment, allowing for safe and effective use of neural networks in various industries.
Committee Members:
Dr. Yimin Yang (supervisor, committee chair), Dr. Amin Safaei, Dr. Fang (Fiona) Fang (Western University)
Please contact grad.compsci@lakeheadu.ca for the Zoom link.
Everyone is welcome.
The Biotechnology PhD candidate, Mahsa Janati will present her research: Experimental Investigation of Water Entry of a Solid Object and Sand Particles
Committee Members: Dr. Amir Azimi (supervisor), Dr. Eltayeb Mohamedelhassan, Dr. Baoqiang Liao, and Dr. Majid Mohammadian (external)
Everyone is welcome
For more information contact Brenda Magajna at phd.ses@lakeheadu.ca